Fully Leveraging Deep Learning Methods for Constructing Retinal Fundus Photomontages.
Kim J. Go S. Noh K. J. Park S. J. Lee S.
Background
Most fundus images have a limited field of view, commonly within an angle of 30 to 50 degrees, depending on the parameters of the imaging equipment. This limitation may not hinder diagnosis of diseases that are localized in a small portion of the retina. However, for retinal diseases mainly affecting peripheral retina, such as diabetic retinopathy or retinal breaks, a wider angle of view is required, because it may be necessary to check the entire retina, including the optic disc, the surrounding of the fovea, and the peripheral regions all at once.
Our Contribution
We present a novel framework for constructing retinal photomontages that fully leverage recent deep learning methods. Deep learning based object detection is used to define the order of image registration and blending. Deep learning based vessel segmentation is used to enhance image texture to improve registration performance within a two step image registration framework comprising rigid and non-rigid registration.
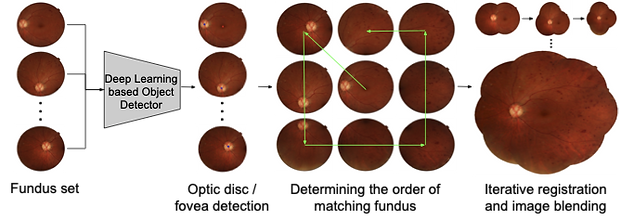
Figure 1. Overview of the proposed framework for constructing retinal fundus photomontages. Through deep learning based object detection, we are able to apply prior knowledge of the fovea and optic disc to determine the optimal order in which to integrate the images into the montage. Deep learning is also leveraged to reduce errors in registration.
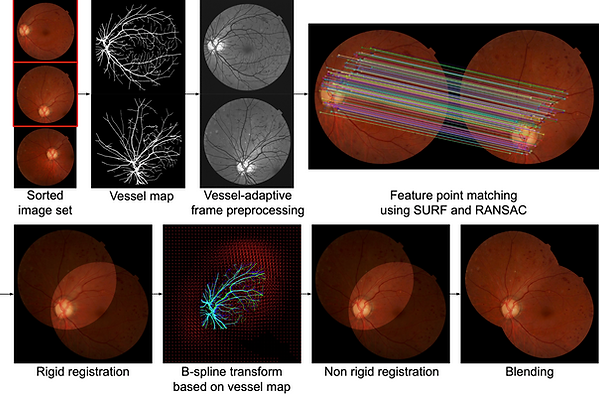
Figure 3. A visual summary of the frame integration pipeline, including a two-step rigid and non-rigid registration method adapted from (Our work published in MICCAI 2019), together with image blending.
Results
Experimental evaluation demonstrates the robustness of our montage construction method with an increased amount of successfully integrated images as well as reduction of image artifacts.
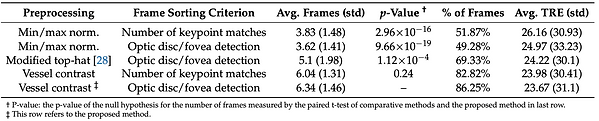
Table 1. Comparison of preprocessing frame sorting methods for constructing a photomontage on 62 image sequences.
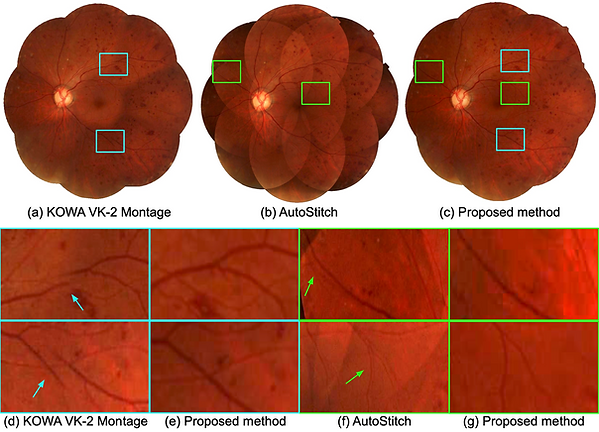
Figure 7. Retinal photomontages constructed by (a) the montage function of KOWA VK-2 [29], (b) AutoStitch [30], and (c) the proposed method, respectively. Local regions highlighted as boxes in (a–c) are enlarged in (d–g). (d) and (f) show the comparison between KOWA VK-2 montage and the proposed method, and (e) and (g) show the comparison between AutoStitch and the proposed method, respectively.
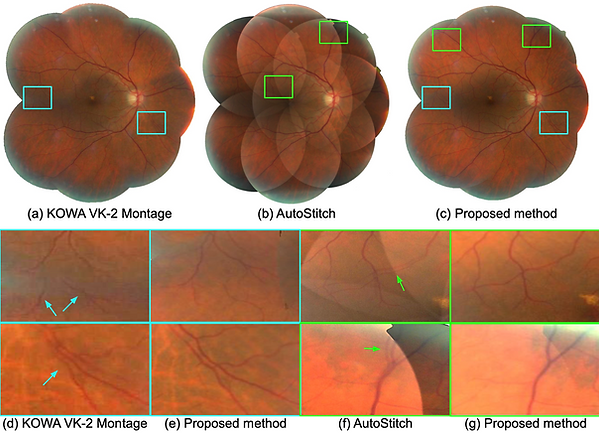
Figure 8. Retinal photomontages constructed by (a) the montage function of KOWA VK-2 [29], (b) AutoStitch [30], and (c) the proposed method, respectively. Local regions highlighted as boxes in (a–c) are enlarged in (d–g). (d) and (f) show the comparison between KOWA VK-2 montage and the proposed method, and (e) and (g) show the comparison between AutoStitch and the proposed method, respectively.
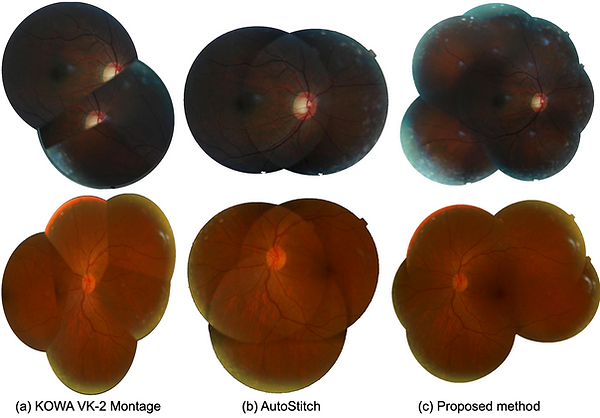
Figure 9. Comparison of Mosaic images constructed by different application. (a) KOWA software. (b) AutoStitch. (c) proposed.
Acknowledgement
This work was supported by the National Research Foundation of Korea (NRF) grants funded by the Korean government (MSIT) (No. 2018R1D1A1A09083241, 2020R1F1A1051847)
Paper
Fully Leveraging Deep Learning Methods for Constructing Retinal Fundus Photomontages.
Kim, J.; Go, S.; Noh, K.J.; Park, S.J.; Lee, S
Appl. Sci., 2021, 11, 1754.
Code
Check our GitHub repository: [Github]
collaboration
Developed in collaboration with researchers from [Seoul National University Bundang Hospital]